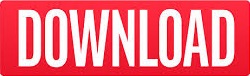
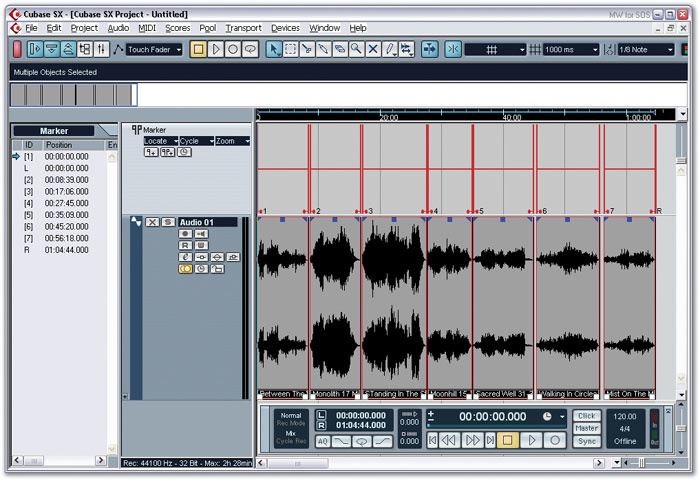
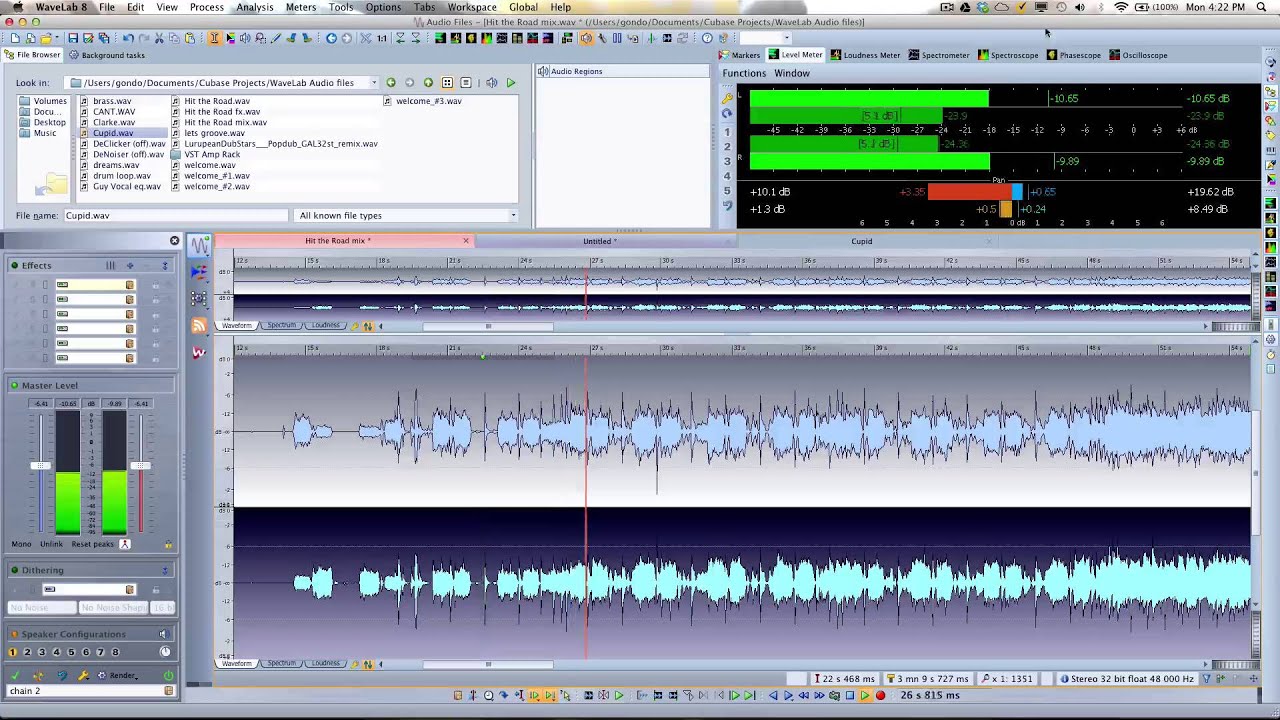
The method calculates the values for "a" and "b" to be used in the formula: Y = a + bX. Linear Regression or Least Squares Regression (LSR) is the most popular method for identifying a linear trend in historical sales data. The two forecast performance evaluation methods are demonstrated in the pages following the examples of the twelve forecasting methods. This recommendation is specific to each product, and may change from one forecast generation to the next. The data in this period is used as the basis for recommending which of the forecasting methods to use in making the next forecast projection. This period of time is called a holdout period or periods best fit (PBF). Both of these performance evaluation methods require historical sales data for a user specified period of time.
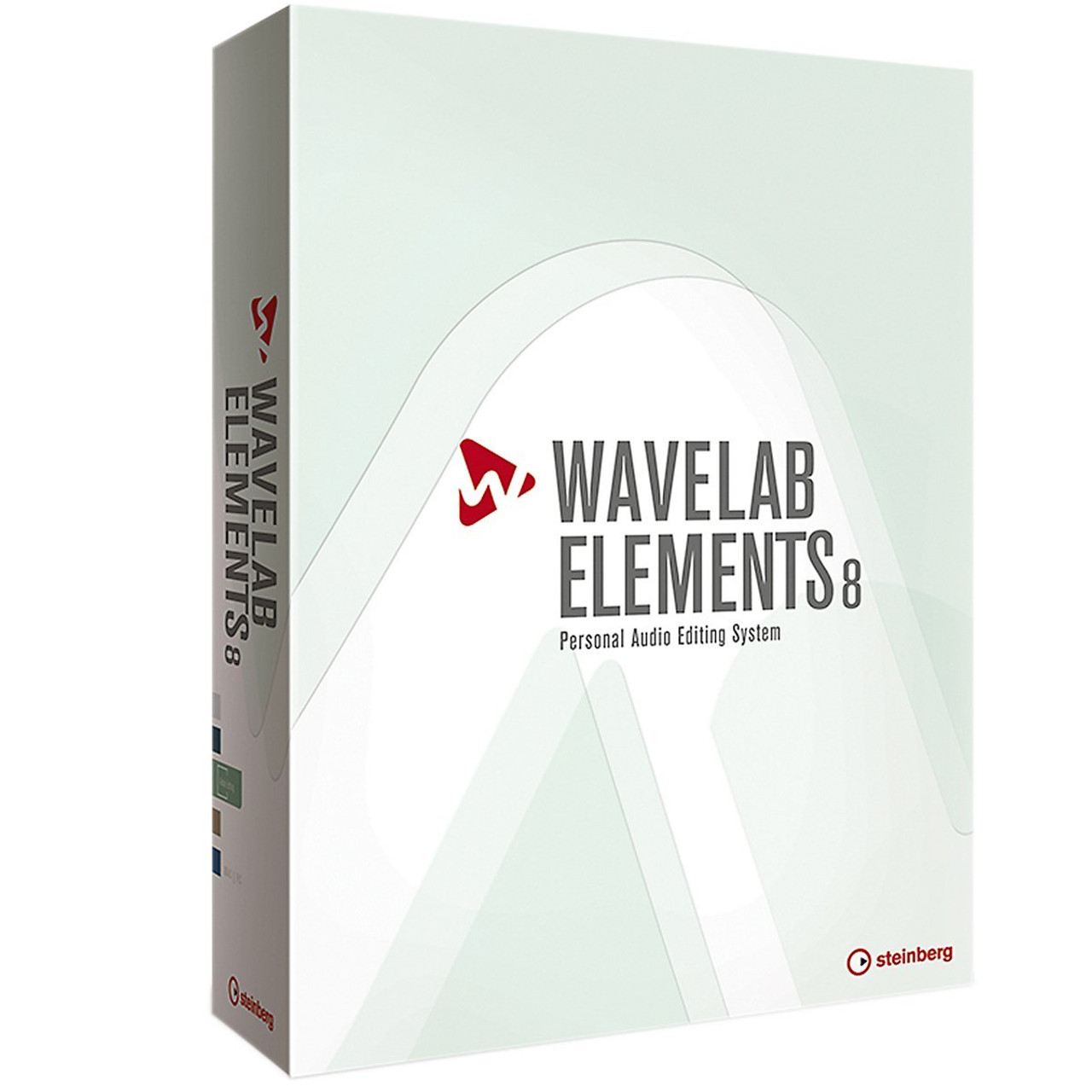
These are Mean Absolute Deviation (MAD) and Percent of Accuracy (POA). You can choose between two methods to evaluate the current performance of the forecasting methods. It is also unlikely that a forecasting method that provides good results at one stage of a product's life cycle will remain appropriate throughout the entire life cycle. A forecasting method that is appropriate for one product may not be appropriate for another product. A.2 Forecast Performance Evaluation Criteriaĭepending on your selection of processing options and on the trends and patterns existing in the sales data, some forecasting methods will perform better than others for a given historical data set.
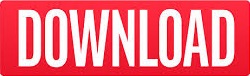